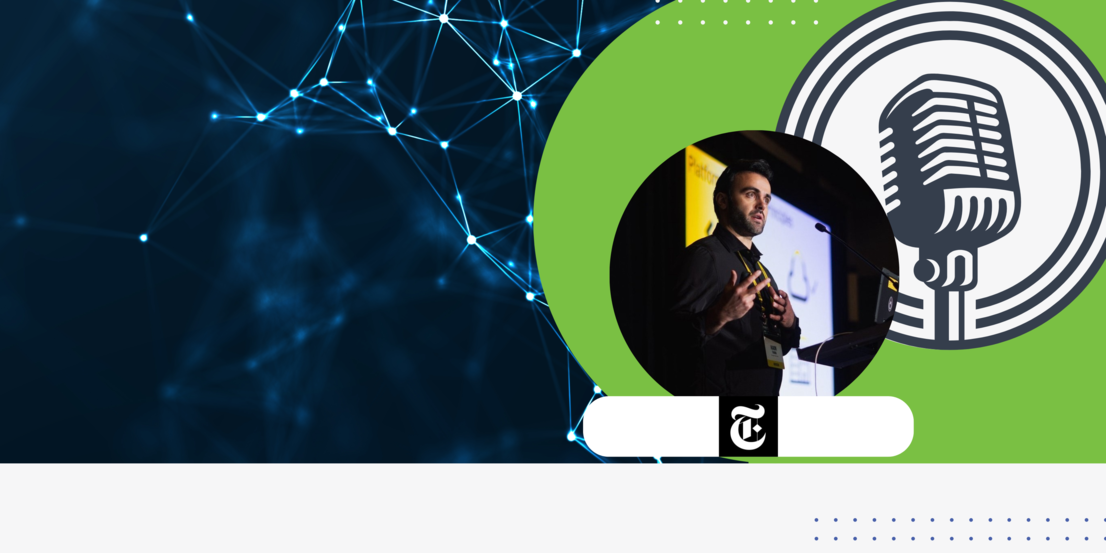
Exploring the future of AI engineering in network security
11 Mar, 20248 minIntegrating Artificial Intelligence (AI) in network engineering stands as a transformative a...
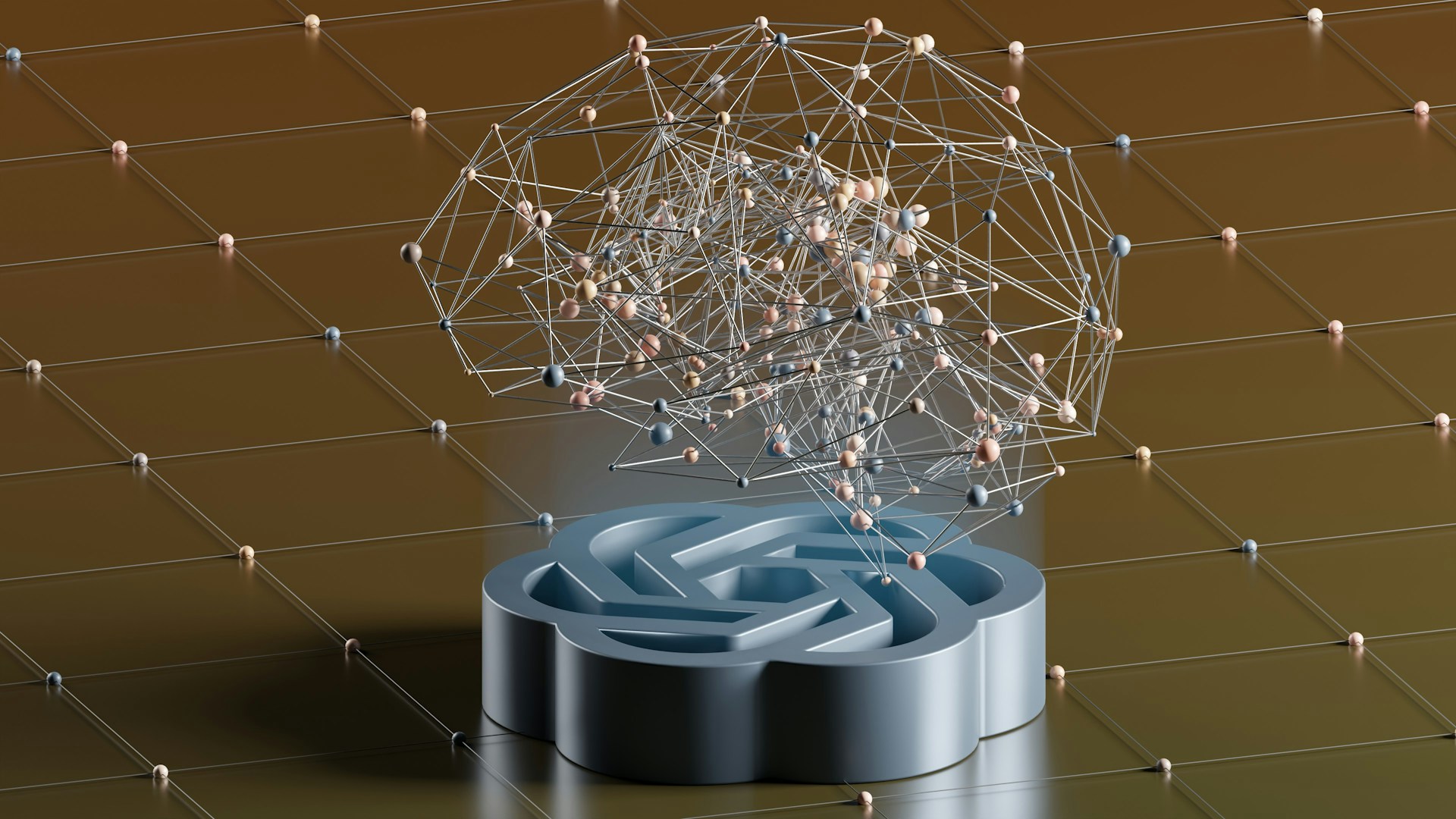
Integrating Artificial Intelligence (AI) in network engineering stands as a transformative approach to the future of tech, significantly impacting network efficiency, scalability, and security. But what is AI in networking, and how is it used? More specifically, how is AI used to support network security, and will AI replace the need for IT engineers? We’ll discuss all of the above in detail as we explore the future of AI engineering in network security.
This guide will explore the following:
- What is AI in networking?
- How is AI in engineering used?
- How is AI in network security used?
- Will AI replace network engineers?
What is AI in networking?
As technology evolves, so do the challenges of managing networks. Therefore, organisations and talent within this space must begin turning to solutions like AI to manage the complexities associated with network engineering.
AI engineering has become a buzzword in the conversation of technological advancements, designed to emulate human-like problem-solving and decision-making capabilities. In the context of networking, AI software is emerging as an essential tool to help address the complexities brought on by the increased number of devices, data, and users across global IT infrastructures.
For organisations dealing with the intricate tasks of network management, turning to AI is increasingly becoming a necessity. While network engineering budgets remain static or decline in the current climate, companies are still seeking efficient ways to navigate the challenges of IT engineering, with AI in networking emerging as a frontrunner to help overcome the complexities of an evolving tech world.
AI in engineering consists of various technologies, all of which uniquely contribute to enhancing the resilience, efficiency, scalability, and security of networks for businesses and service providers.
These key technologies associated with AI engineering include:
- Machine Learning (ML) employs algorithms trained on data to make predictions and execute specific tasks. ML facilitates predictive analytics by continuously learning from patterns and adapting, aiding in proactive network management.
- Natural Language Processing (NLP) allows devices to understand and interpret spoken or written language. In networking, this capability can streamline communication and interaction, facilitating more intuitive and user-friendly interfaces.
- Deep Learning (DL) is a subset of ML that leverages artificial neural networks to simulate the workings of the human brain. This advanced approach to AI engineering allows for nuanced problem-solving and decision-making, enhancing the network's adaptability and intelligence.
- Generative AI (GenAI) utilises DL models to create videos, text, images, and other media formats that closely mimic human-generated content. This innovation is pivotal for content creation and augmentation tasks, providing a more seamless user experience.
These technologies are poised to shape how IT networks are designed and operated. Although AI in networking is still in its relatively early stages, the potential benefits of efficiency, adaptability, scalability, and security could transform future approaches to managing and optimising IT networks.
As organisations continue integrating AI into their network engineering strategies, the future forecasts a symbiotic relationship between AI and networking, where intelligent systems collaboratively navigate the intricacies of modern IT infrastructures.
How is AI in engineering used?
Across various enterprises operating in varying industries, such as healthcare and finance, deploying AI in network engineering brings many advantages in addressing real-world challenges. From identifying time series anomalies and supporting troubleshooting to predicting user experiences and more, the following points outline how AI engineering enhances network security and efficiency:
- AI engineering can identify time series anomalies
AI excels in detecting time series anomalies within networks - issues that occur that deviate from expected outcomes, especially those supporting outdated devices, otherwise known as legacy devices. By correlating various datasets, AI can help network engineers uncover relationships between events that might escape the notice of even seasoned professionals. This ability accelerates the identification of potential network threats or irregularities, enabling proactive measures to be taken.
- AI in engineering can optimise networks
Real-time monitoring by AI enables allocating resources such as bandwidth, processing power, and storage to meet the changing demands of network engineering. AI-driven adjustments in real-time can optimise a network's overall performance, including load balancing, quality of service configurations, and adaptive routing.
- AI in networking can boost scalability
Automation enhanced by AI and ML allows an organisation to automate the deployment of network resources in response to real-time and forecasted tasks and issues. This dynamic scalability ensures network resources align with the evolving requirements of network engineering, allowing optimal performance, responsiveness, and seamless scalability.
- AI in networking supports troubleshooting
AI engineering can monitor complex networks and detect issues by rapidly sifting through extensive datasets. Here, AI helps minimise the number of false positives and assists in detecting the specific components of a network where the fault occurred. Self-healing systems powered by AI can even resolve specific issues without the need for human intervention.
- AI engineering can identify the root cause of an issue
Utilising various data-mining techniques, AI can seamlessly explore vast datasets, aiding IT teams in detecting the root causes of various network issues. Whether related to the type of device involved, operating systems, access points, or switches, AI-driven analysis can accelerate the resolution of network problems. This capability is crucial for efficient event correlation and root-cause analysis.
- AI in engineering can predict user experiences
Instead of solely relying on manual capacity planning, AI can dynamically adjust bandwidth based on historical trends and current usage patterns. This predictive analysis enhances efficiency, ensuring optimal internet performance is granted for users without manual intervention.
Additionally, by comparing historical and current network patterns, AI can identify minor abnormalities before they escalate into significant issues. Based on historical data, predicting user experiences allows AI to model the network effectively, preventing network deterioration or outages. This proactive approach enhances network reliability and reduces the risk of unexpected disruptions.
- AI can enhance customer experiences
Beyond technical improvements, AI can significantly enhance the customer experience. AI minimises network-related disruptions by predicting user behaviour and adjusting bandwidth, ensuring usability remains optimal. Virtual assistants and AI-powered chatbots also offer personalised, context-aware support around the clock, ensuring seamless and responsive customer interaction.
This is especially useful when network engineers need to support a greater number of users than they physically can. AI can help with the more fundamental issues and requests, while human engineers can continue to support more complex issues. Adopting AI can improve customer support, allow an organisation to tackle more problems, and ultimately enhance customer experiences.
- AI can create self-operating networks
AI engineering can also allow IT infrastructures to self-manage, self-drive, and self-correct themselves to maximise a network's uptime and provide precoded actions to resolve issues in real-time. These self-driving capabilities developed through AI engineering reduce the reliance on human intervention and contribute to a more resilient infrastructure where network engineers can focus on broader and more complex tasks.
Ultimately, the integration of AI engineering is a technological upgrade representing a transformative shift in how networks are managed, secured, and optimised for an increasingly interconnected world. But how can AI support network security specifically?
How is AI in network security used?
As the world has become accustomed to remote working and diverse network configurations, the need to protect networks from various threats remains imperative. Of course, a network security engineer can play an essential part in keeping your networks secure. However, their efforts can be enhanced with the help of AI.
Below are the key ways in which AI is used to support network security:
- AI in network security supports threat detection and reaction
Maintaining a threat-aware network is crucial in the modern world of remote work and pop-up network sites. AI engineering can facilitate seamless detection and response to compromised devices, enabling network engineers to react in real-time to potential breaches and network security threats.
The ability of AI in network security to physically locate compromised devices and optimise the user experience highlights the practical benefits of incorporating AI into IT security procedures.
- AI security enhances network risk profiling
AI-driven risk profiling empowers IT engineers with deep network visibility, allowing them to enforce policies at every connection point within a network. This approach protects IT infrastructure by continuously monitoring applications, user connections, and contextual behaviours. It also distinguishes acceptable use from potentially anomalous or malicious activities, forming a secure defence mechanism against network security threats.
- AI in network security is transforming the IT security space
The integration of AI introduces a transformational shift in traditional network security methods. For example, ML algorithms can analyse extensive datasets at speeds surpassing human capabilities, effectively and efficiently identifying patterns and vulnerabilities. Automated tools can also simulate intricate threat patterns, enabling a more thorough review of a network's security posture.
Additionally, AI security can monitor, detect, and respond to security issues as they emerge in real-time. As a result, network engineers can combat problems faster and before they turn into significant issues that put the network and the business in danger of damaging its reputation in the eyes of its users.
- AI security enhances the human touch
AI in network security can also automate responses and enhance human decision-making by providing fast, data-driven insights. Whether speeding up responses or deploying automated self-healing measures, AI is a powerful ally in countering potential threats before they escalate.
In summary, the use of AI in network security can provide businesses with the tools needed to navigate the evolving threat landscape. From rapid threat detection to adaptive defence mechanisms, AI can play a crucial role in fortifying network infrastructure against many security challenges.
Another question that clients and candidates in the network engineering space raise is whether AI will replace network engineers. Scroll to find out.
Will AI replace network engineers?
As the future of AI engineering continues to gain significant ground in network security, the common question arises: Will AI replace network engineers? The simple answer is no - well, not today anyway.
While AI undoubtedly brings transformative capabilities to the field, it's crucial to acknowledge that it is not at the level where it can be used solely to replace human intelligence. Even if it does get to such a level, questions will always be asked whether AI security can be trusted to fully replace humans.
Several factors contribute to the unique value that network engineers bring to the table and why AI in engineering will not fully take over from the human touch. Let's delve into these factors below:
- Engineers have a specialist understanding of network security
Despite their sophistication and benefits, AI security systems lack the nuanced understanding and contextual awareness of network engineering inherent in human decision-making.
Network engineers consider the ethical implications of a decision and apply a human level of intuition that is difficult for AI engineering models to replicate. The human touch in network security remains valuable in addressing intricate security-related issues that require deep expertise in the broader context.
- Humans must supervise AI in network security
AI networking software operates based on algorithms and historical data, and while it can self-learn and evolve, it's still capable of making mistakes. Therefore, human supervision is still necessary and is, in fact, crucial for ensuring the AI security system performs as expected and steers clear of producing unwanted results or false positives.
While AI can be an invaluable tool, the intricate nature of network security engineering still requires human intervention to interpret unique situations and ensure the accuracy of decision-making.
- Network engineers need to support AI with explain-ability
AI algorithms, particularly deep learning models, can lack transparency. This lack of transparency can hinder the AI system's ability to understand its decision-making processes.
Network engineers close this gap in the AI's capabilities with their ability to interpret and explain the rationale behind security decisions, providing a level of transparency and accountability that AI currently struggles to achieve.
- Companies must consider the ethics of AI engineering
Another reason AI in network security still requires a human touch is that AI systems may inadvertently create biases in training data, leading to ethical concerns. With their ethical compass and critical thinking, human engineers are pivotal in mitigating biases and ensuring fair and unbiased network security decisions.
Additionally, users operating within the network will likely still want human interaction when answering their more complex queries and overcoming their more challenging issues. Keeping the human element of network engineering can give users peace of mind that they are dealing with someone who is empathetic, transparent, and unbiased.
- Network engineers will need to be reskilled and upskilled
Finally, integrating AI requires a cultural shift for network engineers, demanding continuous learning to adapt to evolving technologies. As AI in network security is in its relevant infancy, engineers should receive comprehensive training combining traditional IT security knowledge with expertise in AI and ML to develop a system-wide understanding of how they can work with AI - and how they need not fear AI is taking over their role.
Given the rapid advancements in AI security, companies must ensure their network engineers get continuous learning opportunities to stay ahead of the latest tools, techniques, and threats related to AI.
Ultimately, the definitive answer to whether AI security replaces network engineers today is no. While AI brings transformative capabilities, human expertise remains irreplaceable. Engineers provide a nuanced understanding and ethical considerations that AI lacks. This harmonious alliance, combining human strengths with AI capabilities, is crucial for navigating the complex landscape of network security.
Rounding up the future of AI in engineering
The integration of AI in network security is transforming how organisations manage IT networks. Key AI technologies are improving efficiency, scalability, and security, offering multiple benefits in real-world scenarios across various industries.
AI optimises networks, supports troubleshooting, predicts user experiences, and enhances customer support. In network security, it aids in threat detection and risk profiling and transforms traditional security methods, complementing human decision-making.
When it comes to addressing talent concerns, AI engineering is not poised to replace network engineers. Human expertise remains crucial for nuanced understanding, ethical considerations, supervision, explainability, and continuous learning. Ultimately, the future of AI in engineering relies on a collaboration between human strengths and AI capabilities to navigate the evolving world of network management and security.
If you’re looking for help attracting and retaining network security engineers, read our guide on how to attract and retain talent in the network engineering space.
Alternatively, scroll below to see how our recruitment services can help.
Looking to power your future in network engineering?
If you’re looking to embrace AI engineering to support your network security posture but need the talent to do so, we can help. Whether you need permanent or contract network engineers, our enterprise security experts are ready and waiting to connect your organisation with the best candidates on the market.
So what are you waiting for? Get in touch with our network security recruitment team today and power your brand with us.